About GPCRpipe
Basic Theory
G-protein coupled receptors represent the largest and most diverse superfamily of transmembrane receptors in eykaryotic cells, with nearly 800 genes encoding such receptors in the human genome (Bjarnadüttir, et al., 2006). At the interface between the extracellular and intracellular milieu, they are involved in the regulation of nearly every physiological process by converting extracellular stimuli into intracellular responses. Most GPCRs functions are performed by a special group of proteins called G-proteins. G-proteins act as switches for transducting messages from the extracellular space inside the cell, through their interaction with GPCRs (Kristiansen, 2004). G-proteins interact with various effector molecules to immediately change the concentrations of cellular molecules leading eventually to a wide range of cellular and physiological responses (Oldham and Hamm, 2008).
All known members of the GPCR superfamily share common topology: 7 transmembrane á helices, 3 intra- and 3 extra-cellular loops, extracellular N-terminal and intracellular C-terminal. Despite their common architecture and function, GPCRs show important diversity at sequence level. This lack of sequence similarity makes difficult the detection of GPCRs in proteomes, especially, the finding of novel members of the GPCR superfamily.
Here we present GPCRpipe, a pipeline for the accurate detection of GPCRs in proteomes, based on previously published tools and a HMM model especially designed to detect GPCRs.
How to run GPCRpipe?
It is very easy to use GPCRpipe. The user should go to the Run page of the tool and insert in the text box one or more FASTA sequences (up to 100 per run) or upload a text file containing the query sequences, then choose one of the two available methods (AND/OR) using the respective radio button, and, finaly, press the "Submit" button (Figure 1).
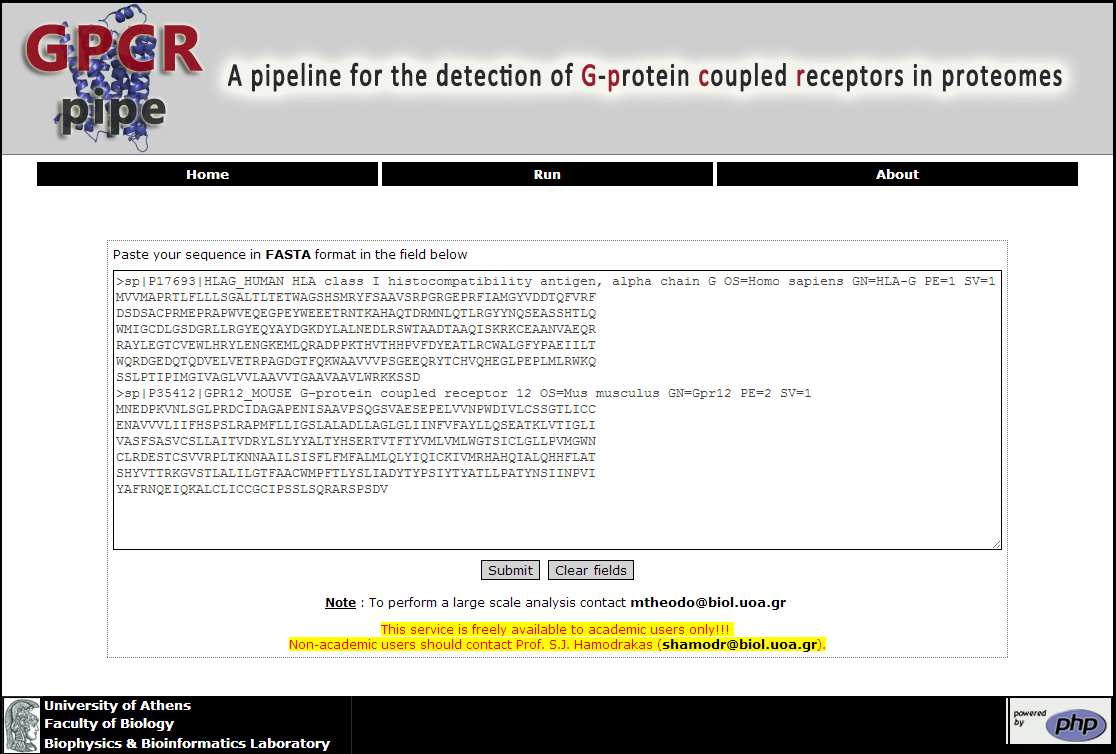
Figure 1. The Run page of GPCRpipe
In a couple of minutes the Result page is return to the user (Figure 2). Here the user may be informed if the submitted sequences are GPCRs or not. For each predicted GPCR, further information is provided. Specifically, the HMM Reliability Score, the GPCR specific Pfam profile accompanied by its limits, the predicted signal peptide via SignalP 4.0 (Petersen, et al., 2011), the coupling specificity via PRED-COUPLE2 (Sgourakis, et al., 2005), the intra- and/or extra- cellular domain profiles accompanied by their respective limits, and, finally, the predicted topology provided by the HMM. On the top of the Result page the text Output file for all the query sequences is provided
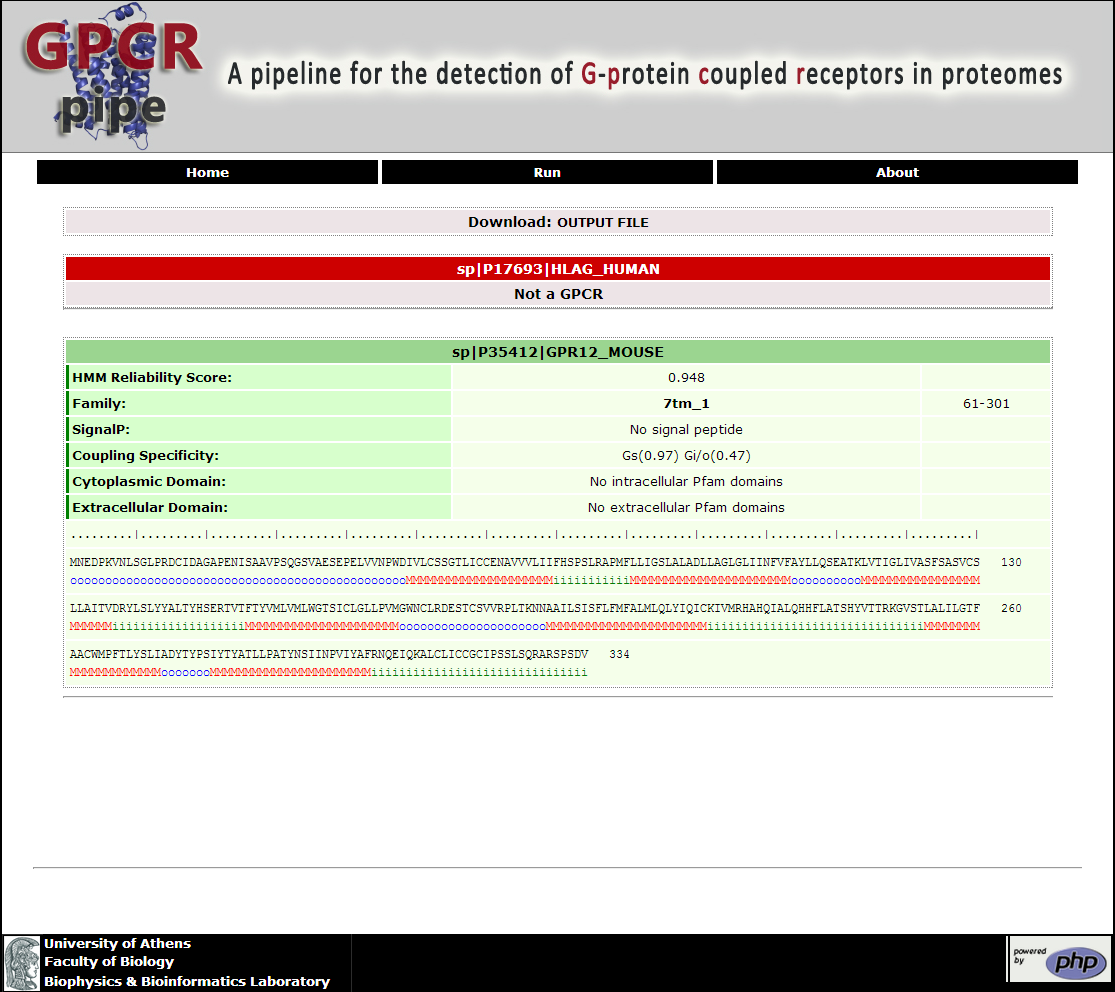
Figure 2. The Result Page of GPCRpipe
How GPCRpipe works?
GPCRpipe consists of two layers (Figure 3). The first layer is the GPCRs' detection, which is the main feature of this pipeline. Two methods are used for the detection of GPCRs in a set of proteins: (a) a HMM especially designed for the detection of GPCRs (Figure 4), which is a modified version of the model used by the HMM-TM algorithm (Bagos, et al., 2006) and (b) a library which consists of 35 PFAM pHMMs (Table 1) which are specific for different families of GPCRs (Punta, et al., 2012). GPCRs are considered all the proteins that are predicted by both methods and only sequences with length greater than 200 amino acids may be detected, as such. The second layer of this pipeline provides features annotation for every predicted GPCR with the use of preexisting tools.
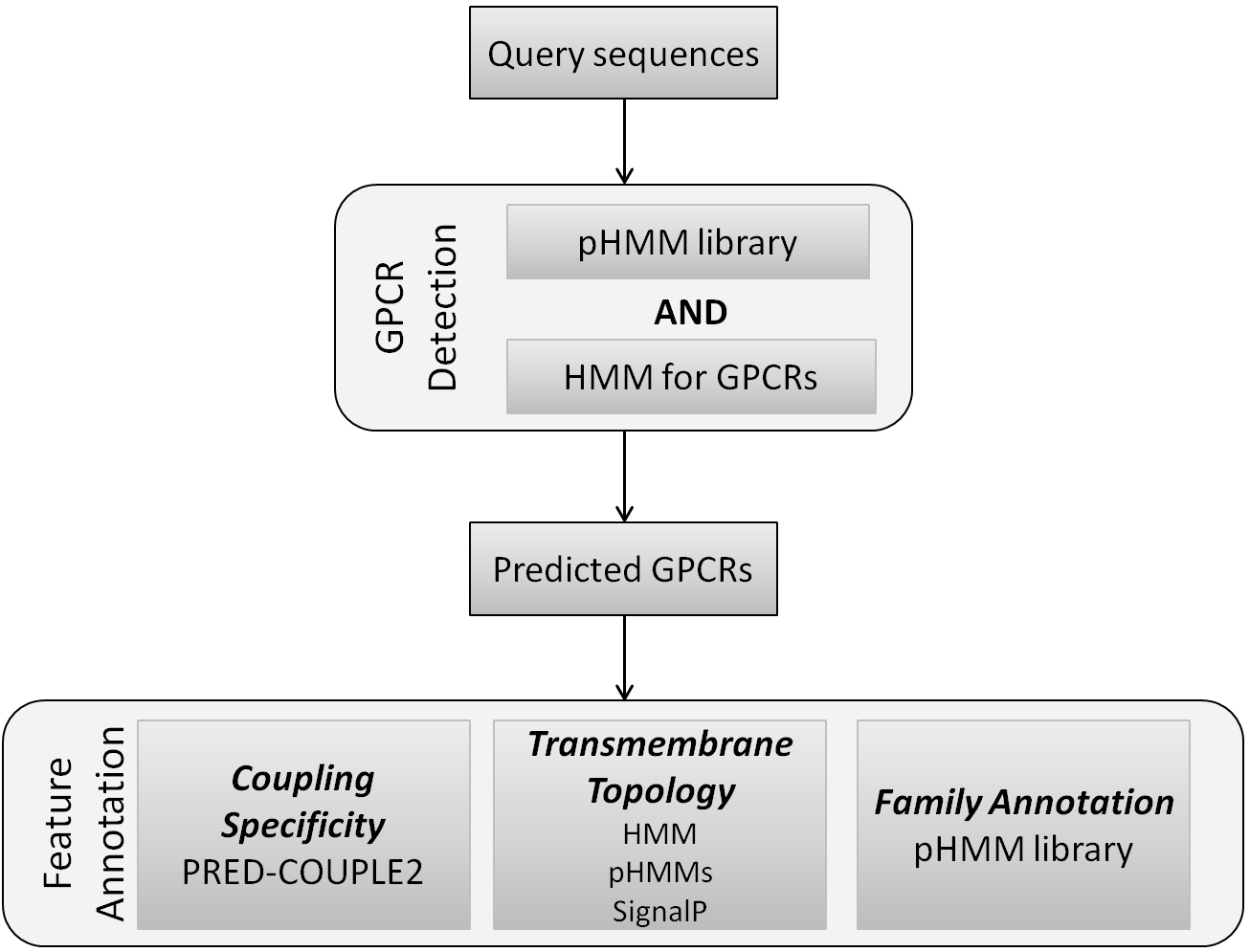
Figure 3. Workflow of the GPCRpipe, consisting of two layers, GPCR detection and Feature Annotation
The model that we used is cyclic, consisting of 114 states, including begin (B) and end (E) states. The model consists of three "submodels" corresponding to the three desired labels to predict, the TM (transmembrane) helix sub-model and the inner and outer loops sub-models respectively. The TM helix model incorporates states to model the architecture of the transmembrane helices. Thus, there are states that correspond to the core of the helix and the cap located at the lipid bilayer interface. All states are connected with the appropriate transition probabilities in order to be consistent with the known structures, that is, to ensure appropriate length distribution. The inner and outer loops are modeled with a "ladder" architecture, at the top each is a self transitioning state corresponding to residues too distant from the membrane; these cannot be modeled as loops, hence that state is named "globular". The model was trained using the Baum-Welch algorithm for labeled sequences (Krogh, 1994) and the decoding was performed using the Optimal Accuracy Posterior Decoder (Käll, et al., 2005).
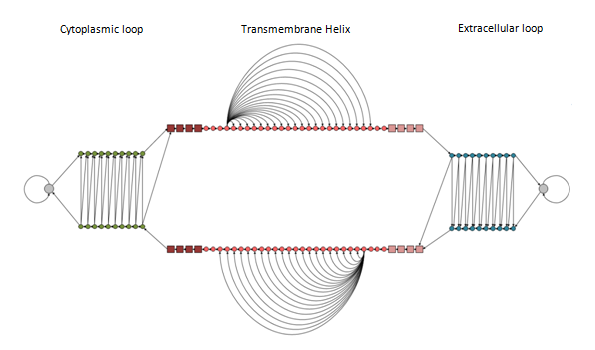
Figure 4. A schematic representation of the model's architecture. The model consists of three sub-models denoted by the labels: Cytoplasmic loop, Transmembrane Helix and Extracellular loop. Within each sub-model, states with the same shape, size and colour are sharing the same emission probabilities (parameter tying). Allowed transitions are indicated with arrows.
Table 1. The 35 Pfam Families (pHMMs) consisting the pHMM library used in GPCRpipe.
In the feature annotation phase, further information is provided for each predicted GPCR:
1. Family annotation using the best hit pHMM from Pfam library (Punta, et al., 2012)(Table 1)
2. Coupling specificity to certain families of G-proteins using PRED-COUPLE2 algorithm (Sgourakis, et al., 2005)
3. Topology provided by (a) the HMM, (b) the occurrence of Pfam profiles with known extra- or intra-cellular position and (c) the signal peptide's position provided by SignalP 4.0 (Petersen, et al., 2011)
The Pfam profiles with known extra- or intra- cellular position were manual gathered. Tables 2 and 3 list the 153 extracellular and the 104 intracellular domain profiles, respectively.
Table 2. The 153 extracellular Pfam profiles used in GPCRpipe.
Table 3. The 104 intracellular Pfam profiles used in GPCRpipe.
Bagos, P.G., Liakopoulos, T.D. and Hamodrakas, S.J. (2006) Algorithms for incorporating prior topological information in HMMs: application to transmembrane proteins, BMC Bioinformatics, 7, 189.
Bjarnadóttir, T.K., et al. (2006) Comprehensive repertoire and phylogenetic analysis of the G protein-coupled receptors in human and mouse, Genomics, 88, 263-273.
Käll, L., Krogh, A. and Sonnhammer, E.L. (2005) An HMM posterior decoder for sequence feature prediction that includes homology information, Bioinformatics, 21 Suppl 1, i251-257.
Kristiansen, K. (2004) Molecular mechanisms of ligand binding, signaling, and regulation within the superfamily of G-protein-coupled receptors: molecular modeling and mutagenesis approaches to receptor structure and function, Pharmacology & Therapeutics, 103, 21-80.
Krogh, A. (1994) Hidden Markov models for labelled sequences. Proceedings of the12th IAPR International Conference on Pattern Recognition. pp. 140-144.
Oldham, W.M. and Hamm, H.E. (2008) Heterotrimeric G protein activation by G-protein-coupled receptors, Nature Reviews Molecular Cell Biology, 9, 60-71.
Petersen, T.N., et al. (2011) SignalP 4.0: discriminating signal peptides from transmembrane regions, Nat Methods, 8, 785-786.
Punta, M., et al. (2012) The Pfam protein families database, Nucleic Acids Research, 40, D290-301.
Sgourakis, N.G., Bagos, P.G. and Hamodrakas, S.J. (2005) Prediction of the coupling specificity of GPCRs to four families of G-proteins using hidden Markov models and artificial neural networks, Bioinformatics, 21, 4101-4106.